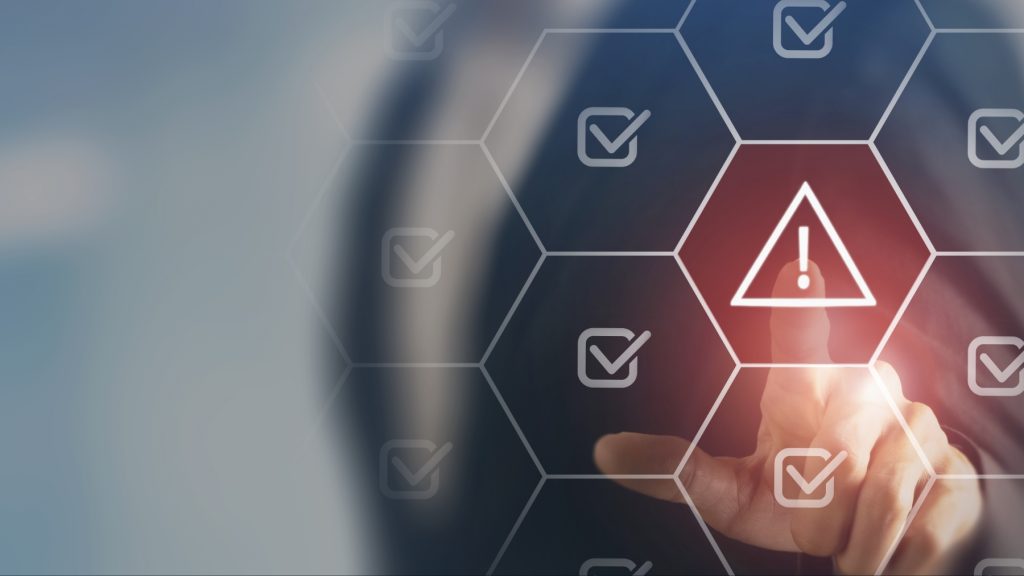
A study published in the scientific journal Nature showed that researchers have developed a new method to detect hallucinations of AI.
Language models such as OpenAI’s ChatGPT are often criticized for having a critical issue known as ‘hallucinations’, which lead them to confidently generate incorrect answers.
The study unveils a new method that could significantly reduce hallucinations made by the AI models. This new technique is said to be 79% accurate, when it comes to detecting correct from incorrect AI-generated answers compared to other well-known methods.
How Does It Work?
The method used involves asking a chatbot to generate multiple answers to one same question, then using different language model to divide these answers by their meanings.
In the next step, researchers will calculate the “semantic entropy,” which measures how similar or different the generated answers are in terms of their meaning. High semantic entropy means that answers generated vary significantly in meaning, showing that the model has a potential to provide inconsistent and incorrect answers.
While low semantic entropy means that answers are similar in meaning, indicating that the model is generating consistent responses even if they are wrong due to other issues.
Although this method uses ten times computing power and tackles only one cause of AI hallucinations, it could pave the way for more reliable AI systems in the future.
Sebastian Farquhar, an author of the study, who is a senior research fellow at Oxford University’s department of computer science, where the research was carried out, and is also a research scientist on Google DeepMind’s safety team, said, “My hope is that this opens up ways for large language models to be deployed where they can’t currently be deployed – where a little bit more reliability than is currently available is needed.”
Outperforming Existing Methods
This method outperformed several existing approaches, including naive entropy and embedding regression. Naive entropy measures the flexibility in the language of answers, focusing on surface-level differences rather than their underlying meanings. Embedding regression involves modifying an AI model on correct answers to specific questions related to a specific topic. While embedding regression ensures accurate responses for certain topics, it also requires large sector-specific training data.
The new method does not rely on this specialized data and works effectively across various subjects, providing a more useful and strong result for detecting hallucinations of AI.
It is somehow true that this technique could change the way AI models interact, making them more reliable, yet its practical application would present some challenges.
Professor of computer science at Princeton University, Arvind Narayanan, emphasized the importance of such research noting that, “it’s important not to get too excited about the potential of research like this,” adding that, “the extent to which this can be integrated into a deployed chatbot is very unclear.”
Inside Telecom provides you with an extensive list of content covering all aspects of the tech industry. Keep an eye on our Intelligent Tech sections to stay informed and up-to-date with our daily articles.