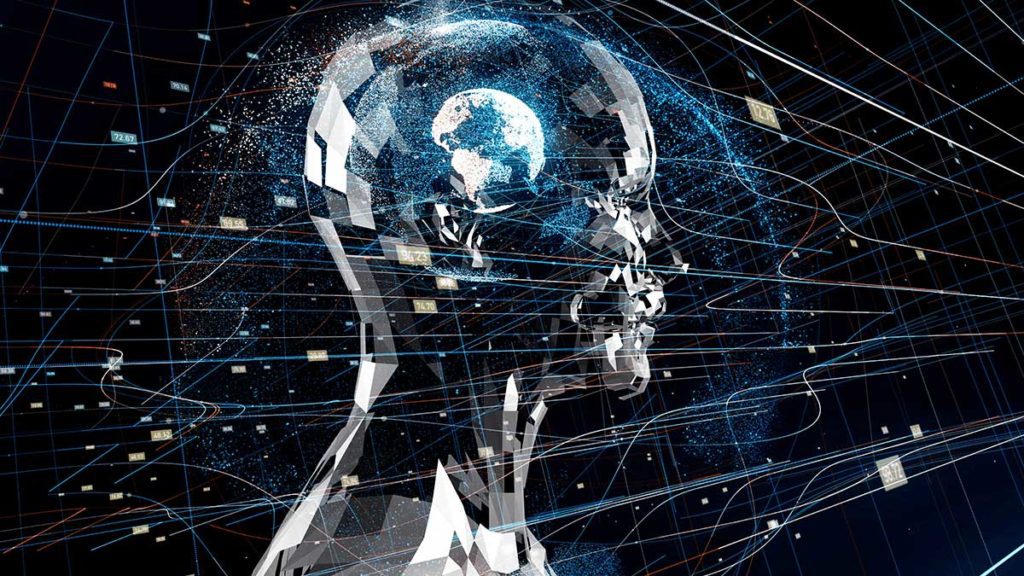
In our digital world, both AI and IoT are significantly changing so many aspects of our lives. The amount of IoT devises that connect to the network is increasing at a rapid rate. By 2025, we are expected to have 41 billion connected devices.
This increasing amount of connectivity means that the data flowing back to the cloud is also on the rise. However, it is not a scalable model to pump all the data back to the cloud for processing. Processing this data at the cloud would push network bandwidth requirements to the limit. Data centers are already struggling to guarantee transfer rates and response times.
Data is now the new oil, however very few companies are able to extract the value from it, despite being surrounded by masses of IoT data. The real value lies in combining datasets from various IoT devices by understanding and interpreting patterns that are able to predict future trends. This is where the potential of AI on the edge comes in and is able to restore true value back to data.
Today, many edge devices have integrated compute power. Many IoT edge device have GPU (graphics processing unit), TPU (tensor processing unit) or VPU (virtual processing unit). As an example, some of the most advance security cameras now feature GPU cards and this enables them to run AI-based image recognition models on the edge itself, instead of sending all the HD video back to the cloud to be processed. Moving processing to the edge ensures for a better response time and a decrease in bandwidth usage.
For an oil and gas refinery that has 1000 edge GPU cameras, one would want to deploy different AI models on different camera nodes based on the location and differences that the cameras were attempting to detect. A red zone in an oil and gas refinery is an area where there is a high chance of fatality due to the possibility of a gas leak. Thus, people entering into this zone need to wear protective gear. Cameras focused on a red zone might detect Health and Safety noncompliance, like not wearing breathing apparatus, and then trigger alarms, thus saving lives.
AI on the edge will enable us to make more sense of data. Its uses are broad and can be applied across numerous industries and verticals, including patient monitoring in healthcare and evaluating the health of crops in farming.
Running these models on the edge must be well thought out. Once the edge has been loaded with AI models, the easy part comes to an end. They should be monitored continuously for performance standards and optimized depending on their scenario.
The diverse nature of connected devices on the edge has come with a unique set of challenges. Remote positioning and deployment of the models and monitoring of the performance is another area that contains huge possibilities. There needs to be a tough mechanism to deploy and perfect the AI models remotely. It is also critical to monitor the health of the hardware.
Monitoring continuously is also a massive job along with the continued deployment, debugging and fine-tuning of AI models on the edge. Few companies have made real progress in this regard.
Security is always a critical aspect that can’t be overlooked. When processing is closer to the edge this puts more stress on having a solid security in and around the edge. Security therefore needs to have a multidimensional approach to ensure the safety of the hardware and software stack. Using AI to power the edge is a potential gold mine that is waiting to be harnessed and has immense potential to bring value to businesses. AI on the edge in the world of IoT will help to provide intelligent and real-time decisions from companies in a cost effective manner.