Machine Learning in Healthcare Prefers Rich People
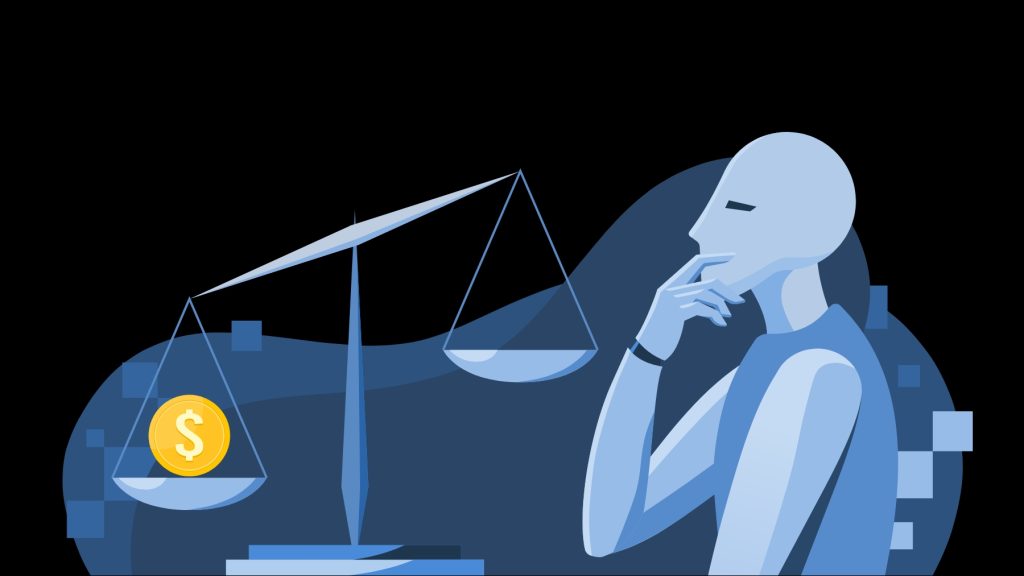
ML Models in Healthcare Biased Towards Well-off
Widely used healthcare machine learning (ML) models for immunotherapy show concerning bias toward higher-income communities.
- This compromises the diversity and the inclusivity of datasets.
- This could lead to inequitable treatment efficacy, defeating the point of personalized immunotherapy.
Rice University computer science researchers found concerning biases in healthcare machine learning (ML) models that are widely used in immunotherapy research.
The study focused on peptide-HLA (p-HLA) binding prediction data, essential for identifying peptides that effectively bind with human leukocyte antigen (HLA) alleles in immunotherapy research.
If a protein is a friendship bracelet, amino acids are the beads. With that in mind, when the body needs to use the protein, it chops it into smaller pieces: peptides. Take insulin as an example. The bracelet (its precursor protein, preproinsulin) is 110 beads long, of which only 51 make up the fragment (the peptide, insulin). So far so good? Awesome.
Now, human leukocyte antigens (HLA) are proteins found on the surface of all your cells. Once manufactured, proteins are transported onto the HLA, forming p-HLA complexes. These complexes tell your immune system (the white blood cells; in this case, specifically T cells) which cells belong to you, and which are strangers. The HLA is specific to the individual. And before you ask, identical twins are indeed the only exceptions. So, yours does not match mine unless we’re identical twins that have been separated at birth. Highly doubtful.
Remember, your body doesn’t like strangers. So, if these patrolling T cells recognize a complex as yours, nothing happens, and everybody goes about their day. However, if they do not, they activate and trigger an immune response against the infected cell.
When we talk about immunotherapy, we refer to boosting, enhancing, or manipulating, a patient’s immune system to fight off diseases, particularly cancer. This is done by estimating the affinity between different peptides and the patient’s specific HLA. The stronger the binding, the stronger the T cells’ reaction to it is. Those estimations are called p-HLA binding predictions.
Let’s say researchers got a satisfactory p-HLA binding prediction for a cancer patient. What they do now is engineer the patient’s own T cells to recognize and attack the tumor’s cells.
Back to the news.
Researchers use certain healthcare ML models to do the estimations. However, Rice University’s computer scientists found that these ML models exhibited a bias towards higher-income communities.
This is a big deal because it means the datasets are not diverse enough to encompass all types of patients. The whole point of immunotherapy is a customized treatment tailored to the patient’s specific needs. A lack of diversity in the dataset would most probably result in inequitable treatment efficacy. We have had enough of them slip through the cracks in the system already.
Beyond patient care, skewing the data toward one end of the spectrum may not accurately predict p-HLA binding. Incomplete data will hinder the healthcare ML model’s job. Some of them, called pan-alleles, claim to be able to predict peptides for HLAs that were never in the dataset in the first place. But to be able to efficiently do that they would need an accurate and diverse pool of data. see the problem. Can you solve a riddle if I omit a key part?
Finally, the most important aspect of this discovery is the further widening of the gap between people. Healthcare was never known to be inclusive. Most medical textbooks, for example, don’t always show what a skin condition looks like on a dark-skinned individual. At one point, a study on a new diabetes medication excluded pregnant women, even though diabetes and pregnancy have a complex relationship. Black women were also excluded from the Women’s Health Initiative study on heart disease. This has led to a lack of understanding of their specific risk factors and treatment needs.
I could go on and on about how the healthcare system has repeatedly failed minorities and the underprivileged. Maybe we could excuse certain instances due to human error. but what’s the excuse for an unfeeling, pragmatic, and logical ML model that has been trained on vast amounts of supposedly diverse data?
Inside Telecom provides you with an extensive list of content covering all aspects of the tech industry. Keep an eye on our Intelligent Tech sections to stay informed and up-to-date with our daily articles.