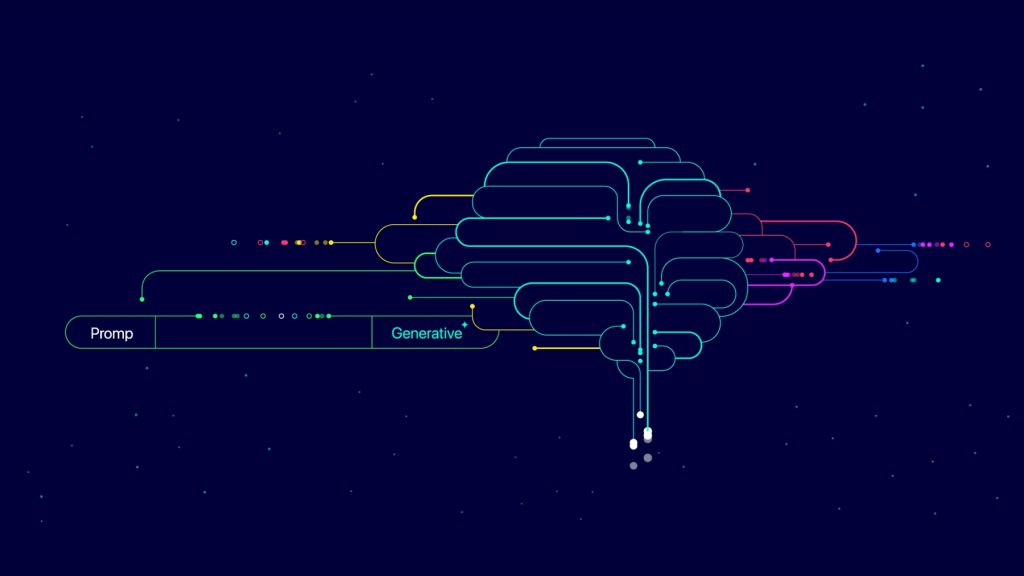
The new TTT architecture could make AI more efficient and consume less power.
- The current standard grows the more data it processes.
- The TTT models remain as they are regardless of the amount of data they process.
A new AI architecture, Test-Timing Training (TTT), promises to solve the AI’s power and computational problems, meanwhile, the ethics of AI use are still unclear.
The world has become obsessed with AI, and it’s rather unsettling. Everyone is using them, and companies are looking for any way to implement them. However, the technology is far from perfect. AI consumes a lot of power and resources, like drinking water, and its abilities are also rather limited still, despite people thinking it will solve all their problems.
Another problem with AI is the lack of defined ethics surrounding its use. However, their absence doesn’t seem to slow down AI development or lessen its power.
TTT Models
Researchers at Stanford, University of California (UC) San Diego, UC Berkeley, and Meta developed the test-timing training (TTT) architecture.
The current architecture is called a transformer. However, it’s inefficient at processing large amounts of data, increasing an AI model’s power demand. These transformers have a “hidden state,” which is a long list of data containing everything a model has processed. Seeing the rate at which AI is growing, this architecture has become computationally challenged. The more data the AI processes, the bigger it gets.
The TTT models address these issues. Instead of a “hidden state,” they have a machine learning (ML) model. Think of an AI within an AI. As this inner model processes data, it encodes it into a set of representative values, referred to as weights. With the TTT architecture, the size of the AI model remains the same regardless of the amount of processed data. This is a significant advantage in scenarios where AI processing power and memory are limited.
Talking to TechCrunch, Yu Sun, a post-doc at Stanford and a co-contributor on the TTT research, explained that one can consider “the lookup table — its hidden state — is the transformer’s brain,”
“Large video models based on transformers, such as [OpenAI’s] Sora, can only process 10 seconds of video because they only have a lookup table ‘brain,’” said Sun. “Our eventual goal is to develop a system that can process a long video resembling the visual experience of a human life.”
Where Are the Brakes?
Since AI’s debut, we’ve seen what people with the worst intentions can do with it. There have been stories of AI-generated explicit content of children, celebrities, and regular people. There has been academic fraud. There has been potential human trafficking disguised as AI paintings on digital market apps like Etsy.
Good things also came out of the AI boom. Teachers’ jobs have become easier to a certain extent. Researching is no longer an extensive scavenger hunt. Small businesses don’t have to spend thousands to even enter competition with rivals.
However, as far as ethics are concerned, the bad far outweighs the good. Governments, experts, and researchers are scrambling to figure out how to use AI without dooming humanity. Meanwhile, companies like Meta are chugging along like the ramifications of an AI whose computing power exceeds anything we’ve seen so far are not their problem, at least for now.
There are also the legalities of AI. But those can be figured out almost naturally once the ethical problems are solved.
Inside Telecom provides you with an extensive list of content covering all aspects of the tech industry. Keep an eye on our Ethical Tech section to stay informed and up-to-date with our daily articles.