There Is No Saving Lives Through AI in Medtech Without Human Touch
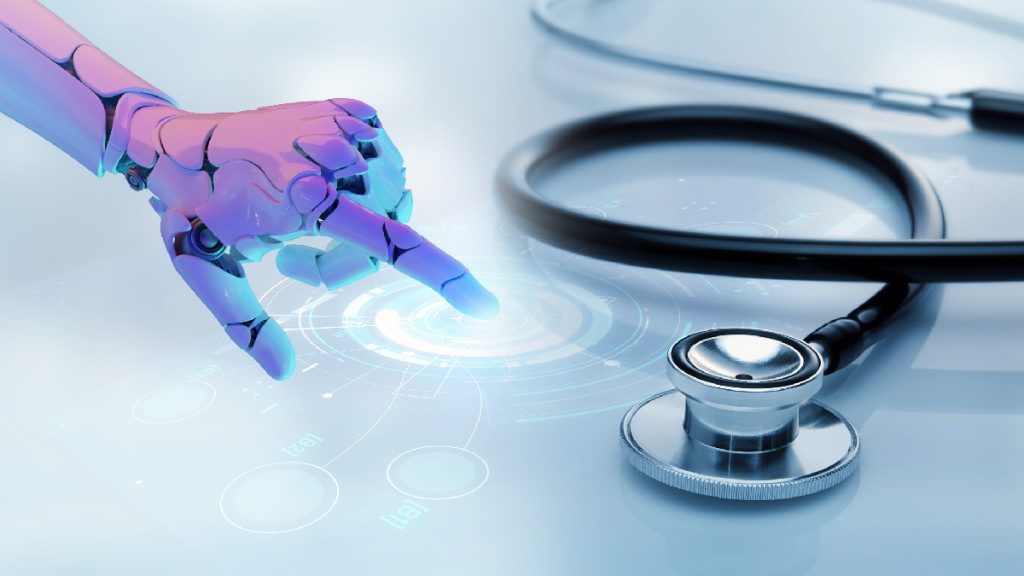
Artificial Intelligence (AI) and Machine Learning (ML) have taken root in every sector imaginable, especially in medical technology (medtech). Through ML and AI in medtech, the rate at which healthcare providers saved lives increased. However, the technology is redundant without the human touch, and the road to recovery is difficult to navigate.
AI means imitating human intelligence processes through machines (i.e., computer systems, etc.). Meanwhile, machine learning describes the algorithm’s capability to learn directly from a large volume of “training data.”
Medtech is an umbrella term that includes every product, service, or solution using medical technology to improve people’s health. Its main tasks are preventing, diagnosing, monitoring, and treating diseases. These innovations are vital to a patient’s quality of life.
As a result, as of October 2022, the Food and Drug Administration (FDA) has authorized around 90 AI-powered or ML-based medical devices, from basic algorithms to more complex ML tools.
Application of ML and AI in Medtech
The main selling point of ML and AI is their ability to analyze an extensive data pool gathered by healthcare organizations that include a slew of images, clinical research trials, and medical claims. In some instances, the technology acts as an extra set of eyes identifying patterns and insights missed by the practitioners due to human error.
Medical Imaging Analysis
AI in medtech is mainly used for case triage. A clinician relies on its help in reviewing images and scans as it is not a victim of human error. AI-powered technology is key to identifying essential insights in prioritizing critical cases. As a result, practitioners avoid mistakes in evaluating Electronic Health Records (EHRs) and establishing concise diagnoses.
A clinical study, for example, includes an intricate set of data. In such a case, AI in medtech is responsible for high-speed analysis and comparison. It aims to identify patterns and out-of-sight interconnections. Consequently, it allows healthcare providers to track crucial information quickly.
AI in medtech makes a radiologist’s job efficient as it presents the relevant issues in an organized summary allowing the creation of accurate and targeted reports for diagnostic purposes.
Medicine Development Cost Decrease
Researchers have been using AI-powered and ML-based machines to predict potential drug uses. The technology refers to databases of molecular structures. Through a convolutional neural network (CNN), AI in medtech hypothesizes if a drug is effective against a disease.
Take AtomNet as an example. The researchers predicted the binding of small molecules to proteins through the analysis of millions of experimental measurements and thousands of protein structures. It successfully identified a safe and effective drug candidate.
This method significantly reduces the cost of drug development and takes off years of research.
Unstructured Data Analysis
Clinics are notorious for having a vast amount of data and medical records on hand, to the point of inorganization. Here is when ML comes in. Machine learning quickly scans EHRs and medical data and provides prompt and trustworthy answers. Patients’ records are often unstructured and difficult to access and interpret. AI in medtech seeks, collects, stores, and standardizes the data, disregarding the format.
This technology takes care of repetitive tasks (i.e., searching, identifying, collecting, and transcribing the solutions from piles of paper-formatted EHRs), freeing up providers’ idle time. Furthermore, it provides fast, accurate, tailored treatment plans.
Complex and Consolidated Platforms for Drug Discovery Building
AI in medtech has the priceless task of identifying and tracing a new drug’s applications, toxic potentials, and mechanisms of action. Such technologies enable the repurposing of existing drugs and bioactive compounds.
Recursion Pharmaceuticals, for instance, can generate around 80 TB of biological data across 1.5 million experiments weekly through the automation and advancement of AI tools. Humans are subject to bias; automation, however, can conclude complex data without interference.
This route of drug identification is favorable as repurposing and repositioning drugs is cheaper than creating them from scratch.
Kidney Disease Forecast
Healthcare providers have a difficult time detecting acute kidney injury (AKI). The disease, unfortunately, can cause patients to escalate quickly and become life-threatening. The estimated percentage of hospital deaths is about 11 percent following a failure to identify and treat patients. Early prediction and subsequent treatment can immensely reduce life-long treatment and the cost of kidney dialysis.
In 2019, the Department of Veterans Affairs and DeepMind Health created a machine-learning tool that predicts AKI up to 48 hours in advance; it identified more than 90% of AKI cases 48 hours earlier than with traditional care methods.
This aspect of AI in medtech quickens the rate of diagnosis and reaction and saves lives daily.
Valuable Assistance Provision
Corti is an AI in medtech assisting emergency medicine staff. It analyzes the caller’s voice, background noise, and relevant data from the patient’s medical history. In case of a heart attack, the AI is tasked with alerting emergency staff. Similar to others of its kind, Corti does have any specific triggers. It trains itself by analyzing thousands of calls to detect crucial factors. The algorithm notices the disparity between background noise (i.e., sirens), oral clues, and patients. A small-scale 2019 study reveals that AI-powered and ML-based models are better at recognizing cardiac arrest calls than human dispatchers. They utilize speech recognition software, machine learning, etc.
Is the Sky the Limit of AI in Medtech? Not Exactly
AI-powered and ML-based tools are versatile in the medical technology field. However, it does come up short in specific departments.
Human Operator
Despite the automation, human supervision is still required in case of malfunction. Take surgery robots, for example. They operate logically and lack empathy. A human doctor may catch behaviors that are vital to the diagnosis.
Social Variables
Unfortunately, robots are yet to be sentient and capable of empathy. They cannot factor social, economic, and historical information into their recommendations. As a result, the AI in medtech may lead the patient to a specific center or clinic based on the hypothesized diagnosis without considering whether it’s within budget.
Privacy Concern
The Health Insurance Portability and Accountability Act (HIPAA) in the U.S. legally binds the healthcare provider into secrecy. The integration of AI and ML in the field could potentially compromise the integrity of the facility.
Potential Unemployment
While AI in medtech does reduce cost and save time, it has the potential to render a job redundant. In such cases, professionals who invested time and money into their education and specialization could find themselves underpaid or, even worse, unemployed. When integrating AI into the medical sector, one must consider the social ramification for the humans involved.
Potential Inaccuracy
As perfect as an algorithm is, it is still artificial. There is still a chance for a misdiagnosis. Such a situation could result in terrible outcomes. A prescription for the wrong treatment could worsen a patient’s state. Furthermore, AI operates on the existing data of diseases and illnesses, and unfortunately, medical information is biased, favoring the majority. Certain cases are not as adequately reported for a minority group as for others.
Security Risks
As AI in medtech depends on data networks, the systems are susceptible to security risks. Cyberattacks can now employ Offensive AI to become more competent and more challenging to predict and prevent.
FDA and AI in Medtech
The FDA has released an extensive list of authorized AI-enabled medical devices. We can notice three key traits of this listing:
- AI devices are popular in Radiology: Three-quarters of authorized submissions are radiology related, with 11 percent being cardiology related. This percentage is due to the extensive imaging and electrocardiogram data available.
- AI devices are not subject to mandatory review: Oddly enough, the majority do not require clinical trials as long as the developers can prove that an equivalent exists on the market. The FDA does not require Academic medical systems to seek authorization for in-house developed algorithms.
- AI devices are getting increasingly complex as the algorithm “learns”: Typically, the AI algorithm remains the same once the device is introduced to the market. The change would require FDA approval. As a result, emerging devices are based on an algorithm capable of assimilating knowledge and learning in contrast to one that remains static once launched.
Wrap-up
Artificial Intelligence AI in medtech is one of the most significant reasons we keep witnessing progression in the medical field regarding apparatuses. AI-powered and ML-based technology can save lives, reduce the cost of research, and cut down on idle time. However, it can also negatively affect the sector. It renders the system sensitive to security risks and open to fatal errors. The key takeaway is that human presence is required and essential for the betterment of patients and the AI itself. A practitioner’s empathy goes a long way toward assuring distressed patients and ensuring their comfort. A relaxed patient means rapid testing, smooth surgeries, and speedy recoveries.
Inside Telecom provides you with an extensive list of content covering all aspects of the tech industry. Keep an eye on our MedTech section to stay informed and up-to-date with our daily articles.