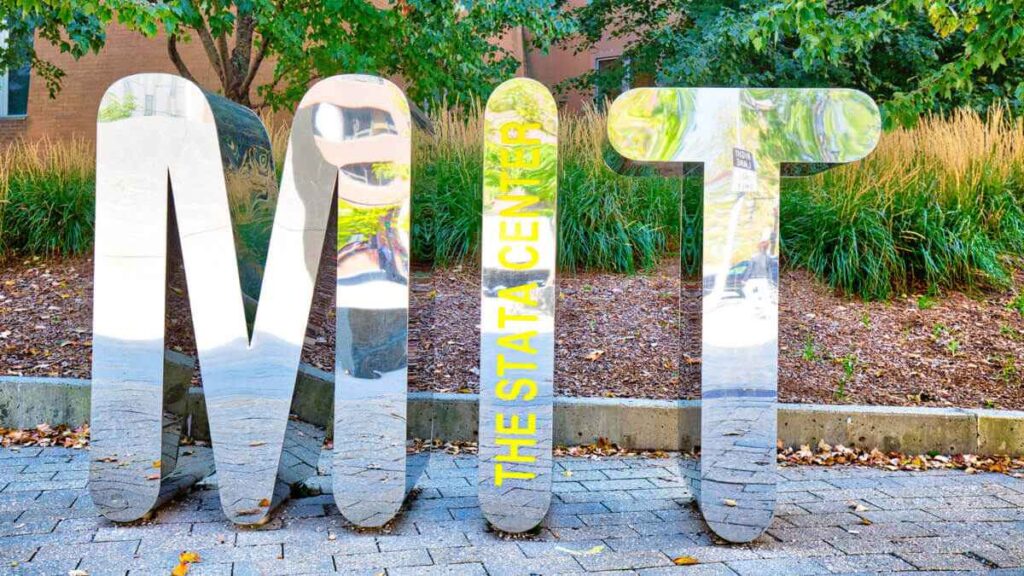
Earlier this week, Massachusetts Institute of Technology (MIT) removed the 80 Million Tiny Images dataset used to train machine learning systems in distinguishing individuals under the pretense that it uses racist and misogynistic labels to identify people.
On Monday, the technology institute published a letter on its Computer Science and Artificial Intelligence Lab (CSAIL) website, apologizing for the removal of their data set, but stressing that its developers will no longer re-upload it into the system.
80 Million Tiny Images dataset creators Antonio Torralba, Rob Fergus, and Bill Freeman revealed that the dataset was massive, and the images were too compacted at 32×32 pixels.
Due to the images’ compression, manual inspection of all demeaning and distasteful content on the dataset will be almost impossible, leading to them taking the last resort and permanently taking down the popular AI dataset. Researchers also urged users to avoid using 80 Million Tiny Images in the long run and remove any downloaded copies.
Initially, the issue had been brought to light by British tech news outlet The Register, which subsequently notified MIT of its findings identifying the problem at hand.
In a paper published, authors Vinay Uday Prabhu and Abeba Birhance revealed that an immense number of images datasets was similar to 80 Million Tiny Images, had a direct association to foul and insulting labels linked to real images. For example, the research discovered over 1,750 images labeled with racial profanities.
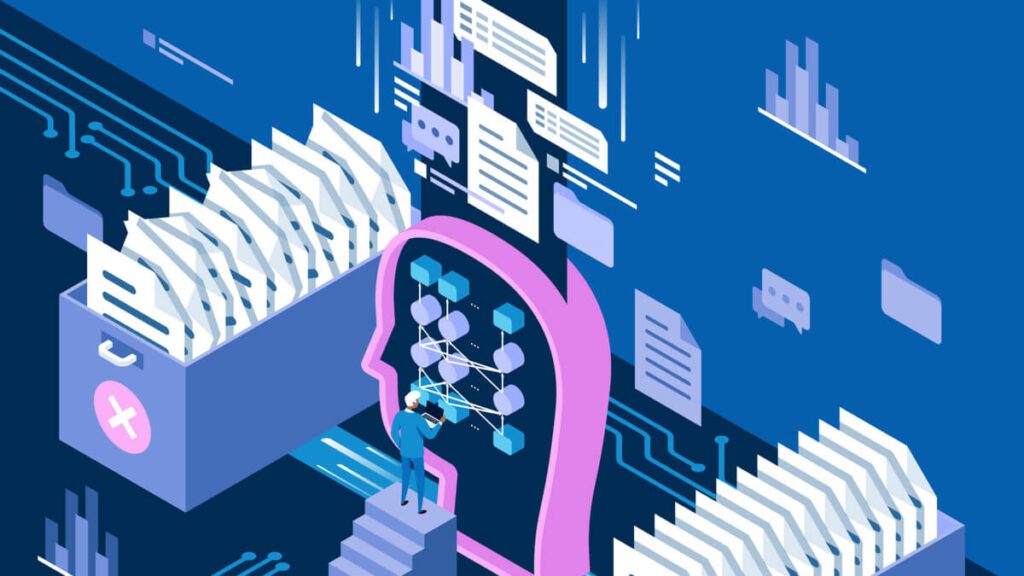
According to The Register, the dataset stamped Black and Asian individuals with insulting racial slurs, women carrying children as whores, in addition to pornographic photos.
A major aspect of the dataset’s AI issue lies in the construction of the dataset and the approach used to build it.
80 Million Tiny Images contains 79,302, 017 images skinned from the internet in 2006, while a different database covering English words was implemented in computational linguistics and natural language processing.
“Biases, offensive and prejudicial images, and derogatory terminology alienate an important part of our community – precisely those that we are making efforts to include,” the dataset engineers said as they expressed their regret to what the dataset led to.
“It also contributes to harmful biases in AI systems trained on such data. Additionally, the presence of such prejudicial images hurts efforts to foster a culture of inclusivity in the computer vision community. This is extremely unfortunate and runs counter to the values that we strive to uphold,” they added.
Since AI is purely based on machine learning and deep learning, any data implemented into the software is nothing but a direct representation of how humans see each other. A machine learning from human behavior only has one choice of functionality: mirroring human behavior and how humans perceive each other.
Not enough education has been released to the public addressing how these datasets are designed, or even the influence AI could carry due to the web’s misleading and misrepresented labeling. Biased datasets could lead to wrongful and immoral consequences when implemented in the training of AI, a technology already released in the real world.
Inside Telecom provides you with an extensive list of content covering all aspects of the tech industry. Keep an eye on our Ethical Tech section to stay informed and up-to-date with our daily articles.